Revolutionizing Cybersecurity: The Power of Malware Detection Machine Learning
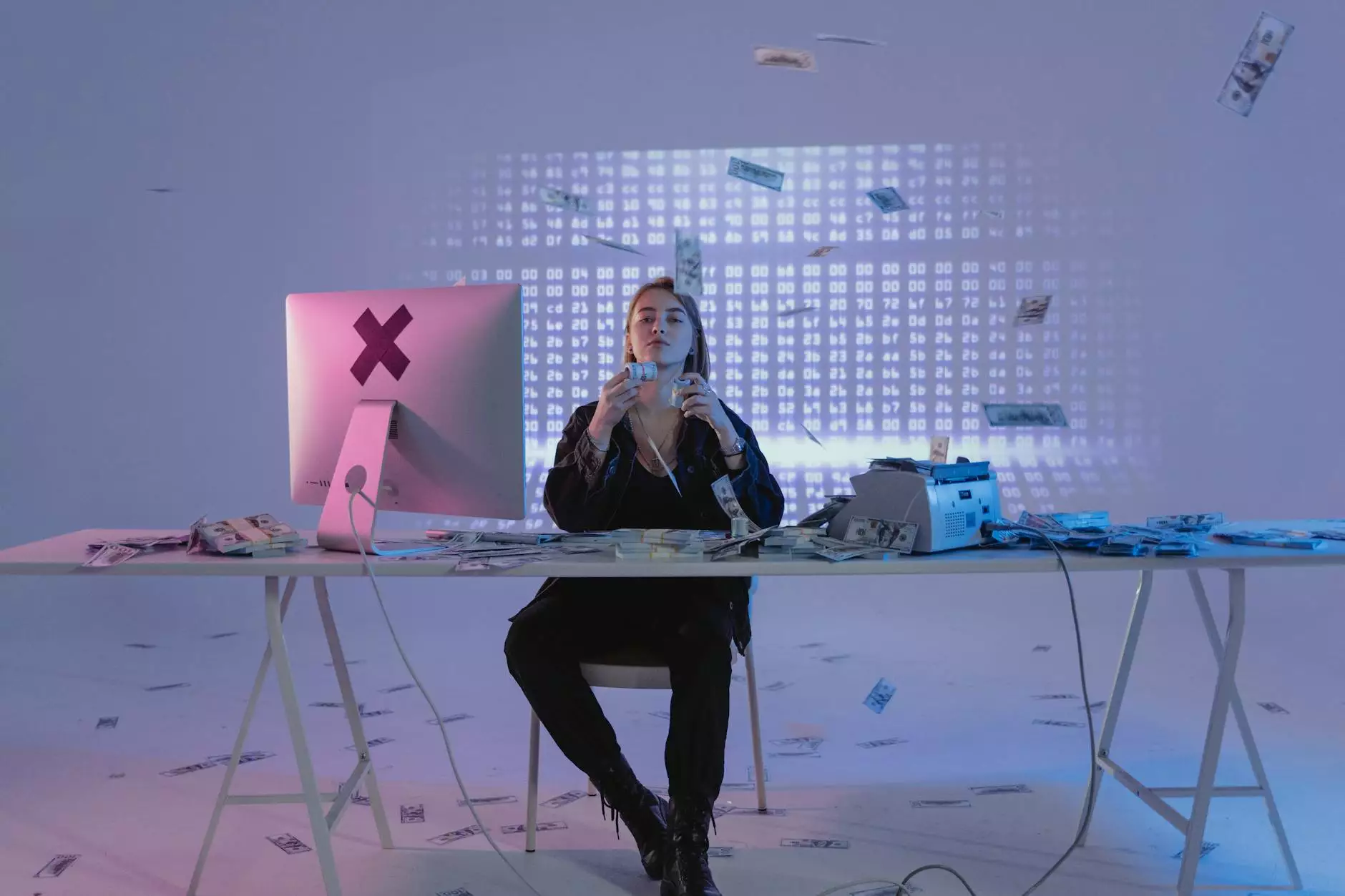
In the digital age where technology is deeply embedded in our daily lives, the threat of malware has become a pervasive issue for individuals and businesses alike. The evolution of cyber threats has necessitated a corresponding evolution in defense mechanisms. Enter machine learning: a transformative approach to malware detection that is reshaping the landscape of cybersecurity. At Spambrella, we pride ourselves on staying at the forefront of technological advancements, particularly in the realm of IT services and computer repair, as well as security systems.
Understanding Malware and Its Implications
Before delving into the specifics of machine learning in malware detection, it’s essential to understand what malware is and the various forms it takes. Malware, short for malicious software, encompasses a range of software designed to harm or exploit any programmable device, service, or network. Common types include:
- Viruses: Malicious programs that can replicate themselves and spread to other devices.
- Worms: Similar to viruses, but they do not require a host program to spread.
- Trojans: Disguised as legitimate software, they can cause harm once installed.
- Ransomware: A type of malware that encrypts files, demanding payment for the decryption key.
- Spyware: Software that collects data and monitors actions without the user's knowledge.
- Adware: Often bundled with free software, it displays unwanted advertisements.
The implications of malware can be severe, ranging from financial loss and data breaches to reputational damage. Organizations, particularly those in IT services, must prioritize effective detection and response strategies.
The Rise of Machine Learning in Cybersecurity
Traditionally, malware detection relied heavily on signature-based methods. This approach involves maintaining a database of known malware signatures and scanning files against these signatures. However, this method has significant limitations:
- It can only detect known threats, leaving organizations vulnerable to new, unknown malware.
- Signature updates are necessary, which can cause delays in threat response.
In contrast, machine learning offers a proactive and dynamic approach to malware detection. By leveraging algorithms that can learn from data, machine learning systems can identify patterns and anomalies that indicate potential threats, even those that have never been encountered before.
How Machine Learning Enhances Malware Detection
Machine learning employs various techniques to enhance malware detection, including:
1. Anomaly Detection
Machine learning algorithms can analyze the behavior of programs and detect deviations from normal behavior. For instance, if a normally benign application starts attempting to access sensitive data or communicates with unknown external servers, the system can flag this as suspicious.
2. Feature Extraction
Machine learning helps in identifying relevant features or characteristics of malware. By focusing on important features, such as file size, creation time, and execution behavior, systems can better distinguish between benign and malicious software.
3. Ensemble Learning
This technique combines multiple models to improve detection rates. By aggregating the strengths of different algorithms, ensemble learning can reduce false positives and enhance the overall accuracy of malware detection systems.
4. Neural Networks
Deep learning, a subset of machine learning, utilizes neural networks to process large amounts of data. These networks can automatically identify complex patterns, making them highly effective for detecting sophisticated malware variants.
Benefits of Machine Learning in Malware Detection
Implementing machine learning for malware detection offers numerous benefits, including:
- Increased Accuracy: Machine learning systems can adapt and learn over time, improving their ability to detect both known and unknown threats.
- Reduced Response Time: With faster detection capabilities, organizations can respond to threats more swiftly, minimizing potential damage.
- Cost-Effectiveness: By automating the detection process, organizations can reduce the need for manual intervention and lower their overall cybersecurity costs.
- Enhanced Threat Intelligence: Machine learning systems can continuously analyze threat data, providing organizations with actionable insights to bolster their security posture.
Case Studies: Successful Implementations of Machine Learning in Malware Detection
Several companies have successfully implemented machine learning for malware detection, yielding impressive results:
Case Study 1: A Major Financial Institution
One of the leading financial institutions in the United States deployed a machine learning-based system to detect fraudulent transactions and malware attacks. By analyzing transaction patterns, the system was able to identify anomalies related to a new malware strain that had bypassed traditional detection methods, leading to a rapid response and prevention of potential losses.
Case Study 2: A Tech Company
A prominent tech company employed machine learning to enhance its existing security infrastructure. By integrating machine learning with its existing signature-based solutions, the company achieved a 30% reduction in false positives and significantly improved its incident response time, protecting sensitive customer data from breaches.
Implementation Strategies for Machine Learning in Malware Detection
Organizations looking to implement machine learning in malware detection should consider the following strategies:
1. Assessing Current Security Posture
Understanding your current cybersecurity framework is crucial. Evaluate existing tools and identify gaps that machine learning could address.
2. Selecting the Right Tools and Platforms
Choose machine learning platforms that best fit your organization's needs, considering factors such as scalability, ease of integration, and support for various data sources.
3. Data Collection and Labeling
For machine learning to be effective, quality data is essential. Collect data on normal versus suspicious behaviors and ensure accurate labeling of known malware to train the algorithms effectively.
4. Continuous Learning and Adaptation
Machine learning models require continual training to stay relevant. Implement a strategy for ongoing learning, incorporating new data and threat intelligence to refine detection capabilities.
5. Collaborating with Security Experts
Engage security professionals who specialize in machine learning. Their expertise can assist in tailoring the system to your organization’s specific needs and ensuring effective implementation.
The Future of Malware Detection with Machine Learning
The future of malware detection machine learning is bright, as advancements continue to emerge. Innovations in AI and machine learning will likely enhance detection capabilities further, making it increasingly difficult for malware to evade detection. Future trends may include:
- Real-time Behavioral Analysis: As computing power grows, real-time analysis of user behavior and application interactions will become more sophisticated.
- Integration with Other Security Technologies: Expect tighter integration of machine learning with other technologies, such as SIEM (Security Information and Event Management) and SOAR (Security Orchestration, Automation and Response) solutions.
- Enhanced Collaboration: Organizations may share threat intelligence more effectively, leveraging machine learning insights across industries to combat emerging threats collectively.
Conclusion
Malware detection is an ever-evolving field, and with the rise of machine learning, organizations have a powerful ally in the fight against cyber threats. At Spambrella, we recognize the importance of leveraging advanced technologies to protect our clients. By implementing machine learning for malware detection, we can not only enhance security measures but also adapt rapidly to an ever-changing digital landscape. Embracing this technology is no longer an option; it is a necessity for any organization seeking to thrive in today's digital economy.